Keynote Speakers
Shafi Goldwasser
Director of Simons Institute for the Theory of Computing and is a professor at UC Berkeley (EECS), MIT (EECS) and Weizmann Institute (CS, Applied Math)
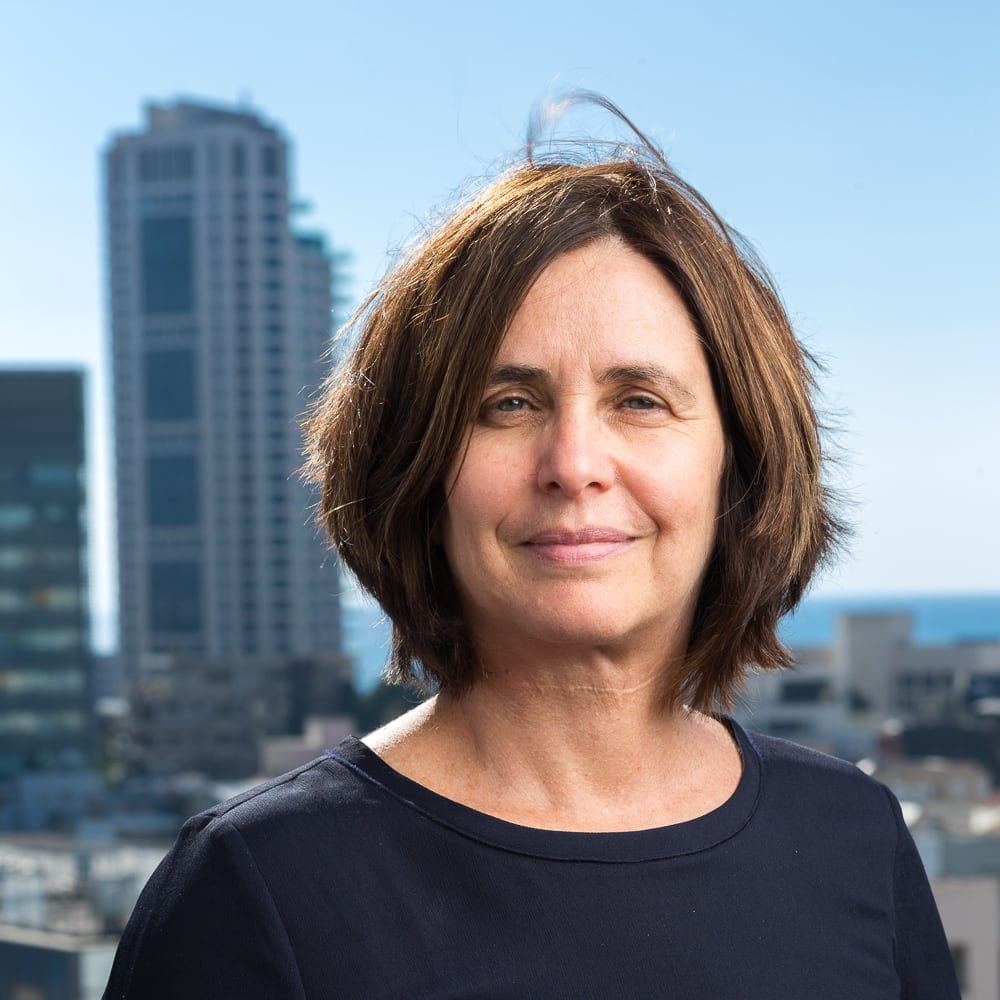
Safe ML: Robustness, Verification and Privacy
Abstract: We will present cryptography inspired models of adversaries in the machine learning landscape and results to address three challenges. These challenges include verification of machine learning models given limited access to good data, training at scale on private training data, and robustness against adversarial examples controlled by worst-case adversaries.
Bio: Shafi Goldwasser is Director of Simons Institute for the Theory of Computing and is a professor at UC Berkeley (EECS), MIT (EECS) and Weizmann Institute (CS, Applied Math). Goldwasser holds B.S. Applied Mathematics, CMU (1979),
Goldwasser’s discoveries include the introduction of probabilistic encryption, interactive
Goldwasser received the ACM Turing Award (2012), Gödel Prize (1993, 2001), ACM Hopper Award (1996), RSA Award in Mathematics (1998), ACM Athena Award (2008), Franklin Medal (2010), IEEE Piore Award (2011), Simons Foundation Investigator Award (2012), BBVA Foundation Frontiers of Knowledge Award (2018), and L’Oréal-UNESCO For Women in Science Award (2021). Goldwasser is a member of the NAS, NAE, AAAS, Russian and Israeli Academies of Science, and London Royal Mathematical Society. Goldwasser holds honorary degrees from many universities.
Mark Sagar
Co-Founder and CEO of Soul Machines and director of the Laboratory for Animate Technologies at the Auckland Bioengineering Institute
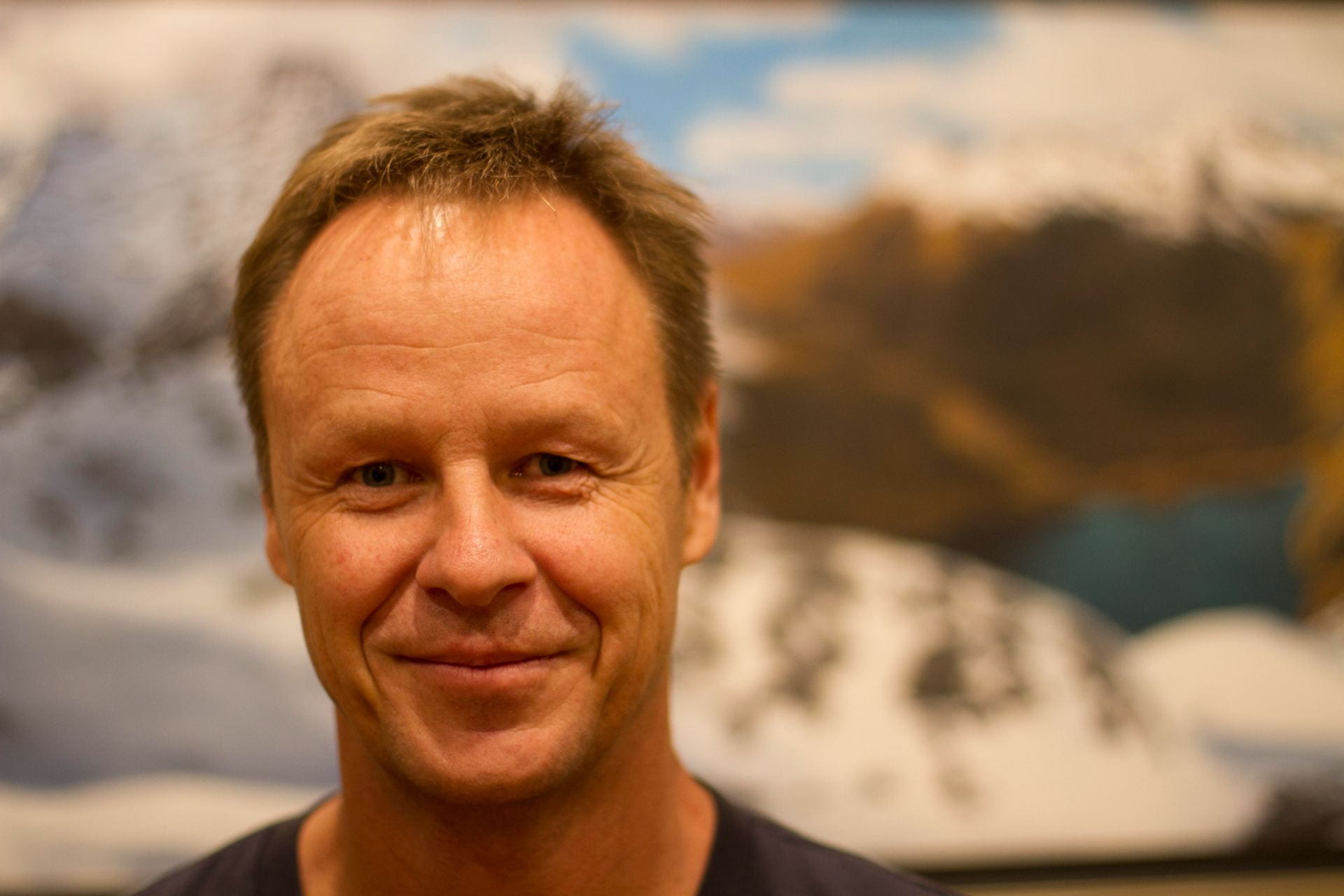
Autonomous Animation
Abstract: Animators create the illusion of life by imagining the behaviours, emotion, motivation and physical embodiment of characters as they interact with other characters and the world. What if a digital character or virtual human could interact with us face to face in real time, and was animated by its own perceptions, emotions and motivations arising from its own experiences and disposition? The talk will discuss some of the challenges in creating autonomously animated virtual humans with virtual brains and nervous systems, capable of highly expressive face to face interaction and real-time learning and emotional response.
Bio: Double Academy Award winner Dr. Mark Sagar is the Co-Founder and CEO of Soul Machines and director of the Laboratory for Animate Technologies at the Auckland Bioengineering Institute.
Mark and his team are bringing technology to life, pioneering the creation of autonomously animated virtual humans with virtual brains and nervous systems, capable of highly expressive face to face interaction and real-time learning and emotional response, to create the next generation of human interaction with artificial intelligence.
Mark has a Ph.D. in Engineering from the University of Auckland, and was a post-doctoral fellow at M.I.T. He previously worked as the Special Projects Supervisor at Weta Digital and Sony Pictures Imageworks and developed technology for the digital characters in blockbusters such Avatar, King Kong, and Spiderman 2. His pioneering work in computer-generated faces was recognized with two consecutive Scientific and Engineering Oscars in 2010 and 2011.
Mark was elected as a fellow of the Royal Society of New Zealand in 2019
Johanna Seibt
Professor, Research Unit for Robophilosophy and Integrative Social Robotics, Aarhus University, Denmark
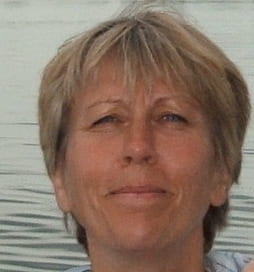
The Aims of Social Robotics
Abstract: The immediate objective of social robotics is to produce embodied artificial agents that can safely perform useful functions in public places, social institutions, or domestic contexts. The focus on physical safety and functionality overlooks, however, that the environments of human social interactions are highly complex symbolic spaces. As neuroscience and
Bio: Johanna Seibt is professor for philosophy at the Department for Philosophy and the History of Ideas, Aarhus University, Denmark. She heads the interdisciplinary Research Unit for Robophilosophy and Integrative Social Robotics, where since 2016 around 30 local and interactional associated researchers from 11 disciplines have collaborated on various projects of HRI research, using the approach of “Integrative Social Robotics” (ISR). The ISR-approach is primarily geared to create culturally sustainable (‘responsible’) social robotics applications but can be extended to other technologies that are designed to operate in the physical and symbolic space of human social interactions (www.robophilosophy.org).
Maria Gini
College of Science & Engineering Distinguished Professor in the Department of Computer Science and Engineering at the University of Minnesota
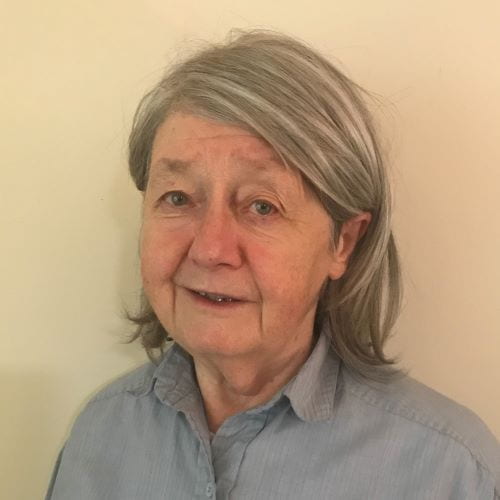
Decentralized allocation of tasks to agents and robots
Abstract: Task allocation and distributed decision-making are common in computer science, robotics, and many related fields. Most algorithms solve the problem in a centralized way as an optimization problem. I am interested in solving task allocation problems with no central authority or only a minimalistic central authority, such as an auctioneer. The methods I have developed work for physical agents that move in 2D space, such as robots or delivery vehicles, as well as methods for virtual agents. I am especially interested in methods that work with temporal and precedence constraints, or for tasks that have costs that increase over time. In this talk, I will present examples of task allocation problems in multiple contexts, some requiring planning in advance and some requiring decisions in real-time.
Maria Gini will give this talk on the occasion of receiving the ACM/SIGAI Autonomous Agents Research Award for her work on AI in the field of robotics and multi-agent systems.
Bio: Maria Gini is a College of Science & Engineering Distinguished Professor in the Department of Computer Science and Engineering at the University of Minnesota. She works on decentralized decision-making for autonomous agents in many application domains, ranging from swarm robotics to task allocation, methods to explore unknown environments, and navigation in dense crowds. She is a Fellow of AAAI, ACM, and IEEE and won numerous awards for teaching, mentoring, increasing participation of women and underrepresented members in computing, and for service. She has published extensively in journals, conferences, and books. She is Editor in Chief of Robotics and Autonomous Systems and is on the editorial board of other journals, including Autonomous Agents and Multi-Agent Systems.
Bryan Wilder
Schmidt Science Fellow at Carnegie Mellon University and Harvard School of Public Health
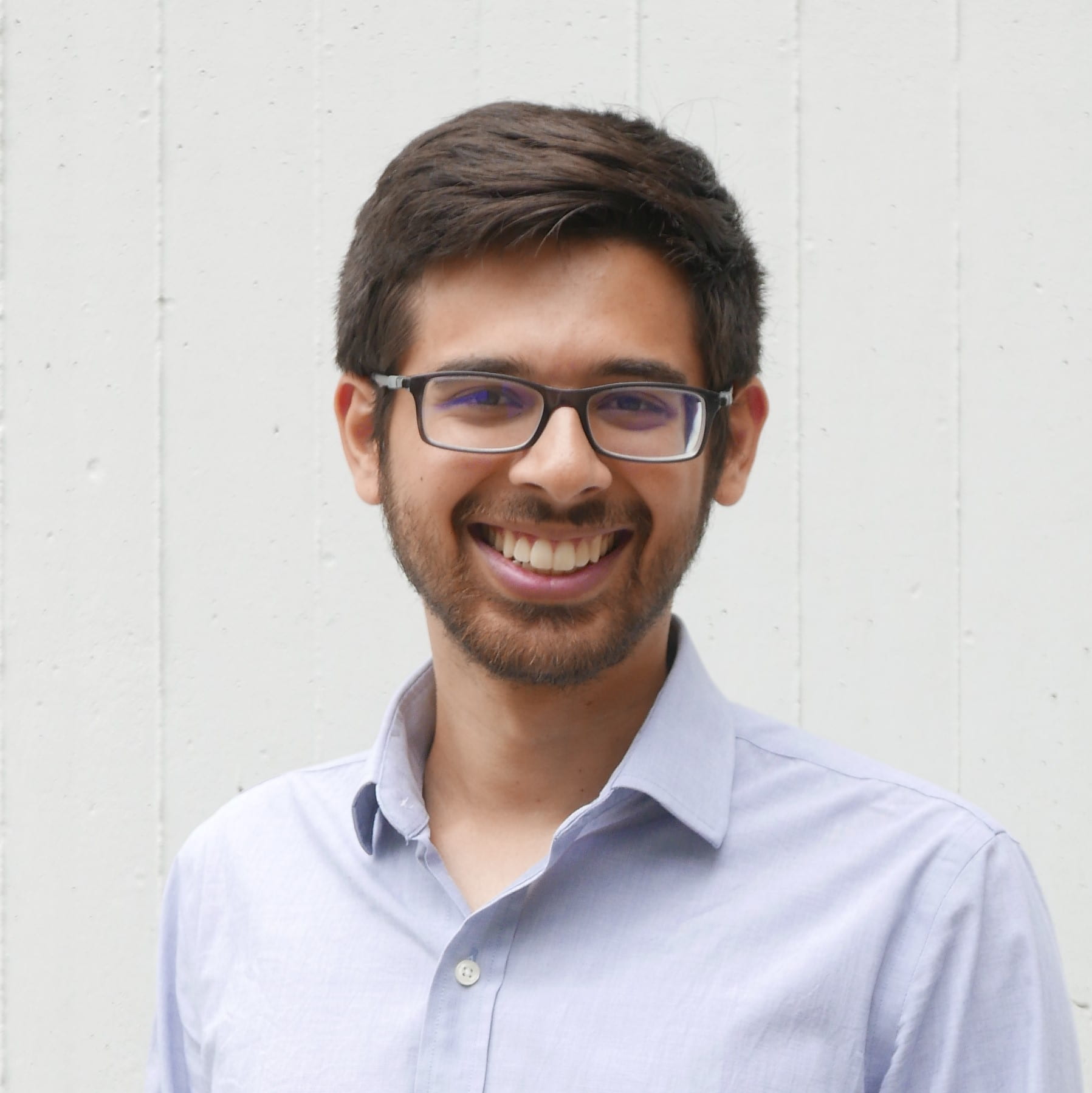
AI for Population Health: Melding Data and Algorithms on Networks
Abstract: As exemplified by the COVID-19 pandemic, our health and wellbeing depend on a difficult-to-measure web of societal factors and individual behaviors. My research aims to build computational methods which can impact such social challenges. This effort requires new algorithmic and data-driven paradigms which span the full process of gathering costly data, learning models to understand and predict the interactions of many agents, and optimizing the use of limited resources in interventions. In response to these needs, I will present methodological developments at the intersection of machine learning, optimization, and social networks which are motivated by on-the-ground collaborations on HIV prevention, tuberculosis treatment, and the COVID-19 response. These projects have produced deployed applications and policy impact. For example, I will present the development of an AI-augmented intervention for HIV prevention among homeless youth. This system was evaluated in a field test enrolling over 700 youth and found to significantly reduce key risk behaviors for HIV.
Bryan Wilder will give his talk on the occasion of receiving the Victor Lesser Distinguished Dissertation Award.
Bio: Bryan Wilder is Schmidt Science Fellow at Carnegie Mellon University and Harvard School of Public Health. In Fall 2022, he will join CMU as an Assistant Professor in the Machine Learning Department. His research focuses on the intersection of optimization, machine learning, and social networks, motivated by applications to public health. He was previously supported by the NSF and Siebel Fellowships and his work has been a finalist for best paper awards at ICML, AAMAS, and the INFORMS Doing Good with Good OR competition.